Mixture of Experts architektúra
A Mixture-of-Experts (MoE) egy gépi tanulási architektúra, amely a "felosztás és uralkodás" elvét követi. Az alapötlet az, hogy egy nagy modellt több kisebb, specializált almodellre – úgynevezett "szakértőkre" – bontanak, amelyek mindegyike egy adott feladatra vagy adathalmaz-részletre specializálódik.
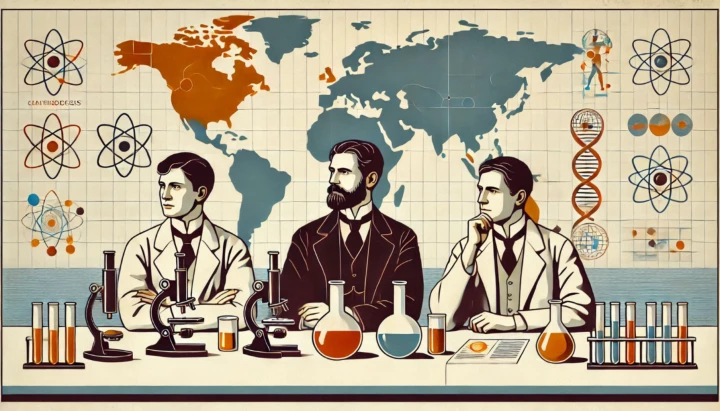
Egy választó kapu (gating) hálózat dönti el, hogy az adott bemenethez mely szakértő(ke)t aktiválja, így a számítási erőforrások csak a releváns részeket használják.
Fő elemek:
- Szakértők (Experts): Ezek különálló alhálózatok, amelyek egy adott részfeladatot oldanak meg.
- Gating hálózat: Egyfajta "forgalomirányító", amely meghatározza, hogy az adott bemenetet mely szakértők dolgozzák fel.
- Szakértő aktiváció: Csak néhány szakértő aktiválódik egy adott bemenet feldolgozásához, így jelentős számítási és memóriahatékonyság érhető el.
Működés szemléltetése:
Képzelj el egy csapat szakembert (pl. matematikust, nyelvészt és programozót), akik együtt dolgoznak egy komplex problémán. Amikor egy kérdés érkezik, például "Írj egy programot!", a csapatvezető (gating hálózat) a programozót választja ki a feladat megoldására. Ha viszont egy matematikai problémát kapnak, akkor a matematikus kerül előtérbe. Így mindenki csak azon dolgozik, amiben a legjobb.
Előnyök:
- Hatékonyság: Csak a releváns szakértők aktiválódnak, ami csökkenti a számítási költségeket.
- Skálázhatóság: A modell könnyen bővíthető további szakértőkkel anélkül, hogy az egész rendszert újra kellene tanítani.
- Specializáció: Az egyes szakértők jobban tudnak alkalmazkodni az adott feladatokhoz.
Példa:
Egy modern MoE modellben, mint például a Mixtral 8x7B-ben, minden réteg 8 szakértőt tartalmazhat, amelyek közül csak 2 aktiválódik minden bemenetnél (top-k routing). Ez lehetővé teszi, hogy egy 56 milliárd paraméteres modell hatékonyan működjön úgy, mintha csak 14 milliárd paramétert használna egyszerre. Az MoE tehát különösen hasznos olyan nagy modellekben (pl. nyelvi modellekben), ahol a számítási költségek és memóriaigények optimalizálása kulcsfontosságú.